As IoT grew in popularity, there was an influx of smart devices linked to the Cloud. This rise, however, produced network congestion because networks were not built to manage such high demand. Security was a concern since sensitive data was being exchanged via networks. As storage and processing requirements grew, edge computing evolved.
Edge computing refers to the practice of deploying computers and data storage closer to the data source. This eliminates the need for data to be processed in a faraway data center and provides advantages such as faster insights, enhanced bandwidth availability, and quicker response times.
While edge computing addressed some of the problems, things became complicated when the expansion of sensors and smart devices created traffic bottlenecks that could have improved performance. The solution to this challenge eventually emerged as edge machine learning.
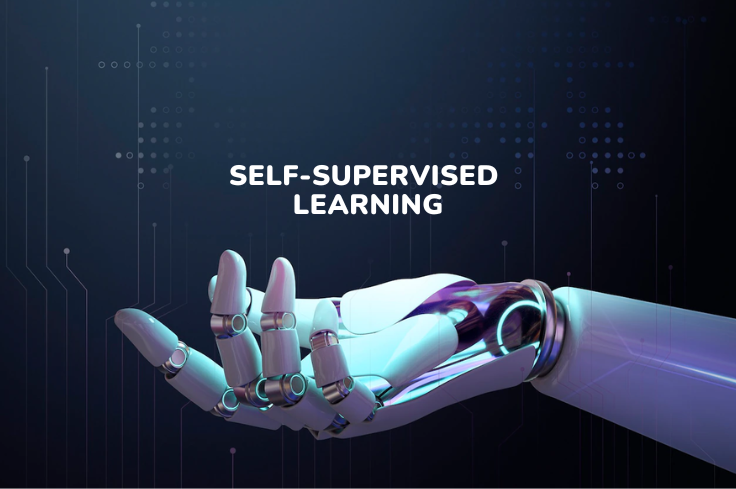
Edge machine learning is an approach that allows smart devices to process data locally on the device, either using local servers or machine learning algorithms. The term ‘edge’ refers to the processing these deep learning or machine learning algorithms perform at the device or local level. Even though some data is delivered to the Cloud via these edge devices, this decreases reliance on cloud networks. Yet, the ability to analyze some or all of the data locally enables the filtering of the data sent to the Cloud. It also makes real-time data processing and response possible.
Edge machine learning employs both deep learning and machine learning methods to enable local data processing, depending on the application. Unlike typical data processing computers, edge machine learning devices may process incoming data at the source. It then selects which data need more robust algorithms for processing in the Cloud and which data can be processed locally.
Let’s take the virtual assistant Siri as an example. When you ask Siri to tell you a joke or story, the data is processed locally on the device and does not need to be processed on the Cloud. This is because the jokes or games are kept locally on the device.
The device executes the command without interfering with the operation of the Cloud network. But, when you ask Siri for the weather, the device searches an external source in the Cloud for the information. We can also use edge machine learning to analyze massive data volumes in real-time, which is currently not possible with traditional cloud-powered devices but is critical for applications such as medical devices and driverless vehicles.
Edge machine learning alleviates security concerns associated with storing personal user data on the Cloud. The Cloud’s load is also considerably lessened. This also minimizes the likelihood of DDOS assaults because edge devices decentralize data storage. Edge machine learning also improves data privacy and security since the algorithms can be trained to ignore critical data areas.
Because data is handled locally in algorithms stored on a hardware device, edge machine learning increases system reaction times. Edge machine learning deals with resource constraints, processing capacity, memory issues, response times, and Cloud security concerns.
Why is Edge Machine Learning Important?
Machine learning is continually growing in order to provide more accurate predictions and uncover critical trends more quickly. Edge machine learning is becoming more important currently because:
• By evaluating customer data more quickly makes it easier to detect data anomalies such as malfunctioning devices or data anomalies.
• It addresses the growing requirement in AI and machine learning projects to optimize workloads from training and inference. By relieving edge devices’ central processing units of complicated and heavy mathematical work, edge machine learning makes it faster, cheaper, scalable, and more power-efficient.
• As the Internet of Things uses cases become more tangible in everyday life, the demand for intelligent decision-making at every step in the infrastructure stack will grow.
• The growth of self-improving products necessitates using cutting-edge machine learning to create individual enhancements.
• Security issues are growing, and feature extraction utilizing neural network partitioning will become critical for safely transmitting data over the network, balancing workloads and latency, and efficiently managing bandwidth needs.
• The ability to recognize complicated events by processing massive data sets in real time is improving.
• It is becoming increasingly necessary to optimize and reduce network costs while also providing offline availability by storing intelligence locally.
• Staying ahead of the regulatory curve requires enabling improved compliance and enhancing security posture by acquiring and processing confidential and essential data locally.
In conclusion
Although edge machine learning is a rapidly changing field, it has the potential to become critical for next-generation solutions. It is an interesting technology that increases predictive skills and performance by analyzing data and sending messages in real time, enhancing responsiveness. It is only a matter of time until edge machine learning becomes a mainstream technology and part of our daily life.
Furthermore, In the future hospitals and assisted living facilities may develop Edge ML-based systems to monitor things like patient heart rate, glucose levels, and falls (using cameras and motion sensors). These technologies have the potential to save lives, and if the data is analysed locally at the edge, employees would be notified in real-time when a speedy response is required to save lives.
Edge ML is an intriguing new technology that is still being discussed and researched. It will only be a matter of time before Edge ML-powered gadgets (like the Internet of Things) become commonplace. And what a thrilling time it will be.