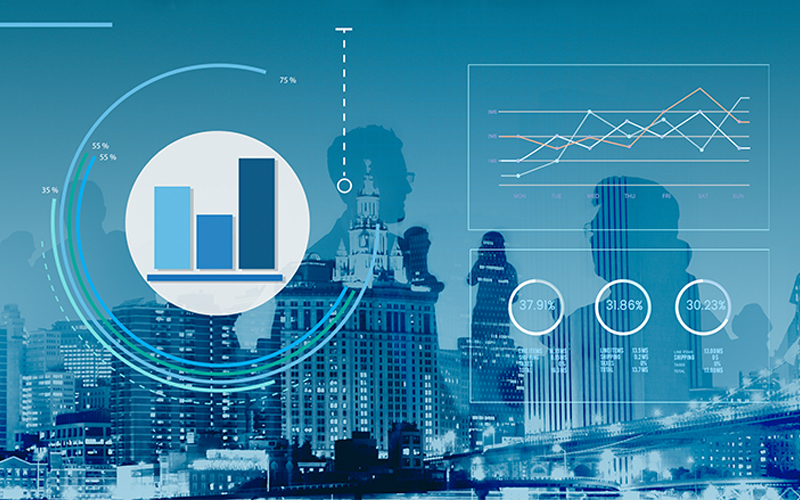
How would you feel when you’d be met by alerts for sales on items you didn’t want to buy and pages you couldn’t navigate? In this hyper-personalized era, customers expect personalized recommendations instead of aimlessly wandering the aisles. Be it a solution from Alexa or impressive BI or product recommendation, all are the results put in by Data Engineers, and it’s tough to imagine an industry that has not been disrupted by Data engineering yet.
No matter what size the business is, it usually sees data in many forms like profile visits, clicks on the websites, bounce rates, marketing ads, social media posts, and much more. Managing, collecting, storing, and analyzing this data at scale is what data engineering has to do with. Data engineers set up the data warehouse, data framework, and data pipelines that can further assist data scientists to interpret and convert valuable decisions and strategies.
Certainly, data engineering is critically important in this data age and its megatrends will have a major impact across the industries. Here are the top five data engineering trends to watch out for:
- Transitioning to Cloud
More and more companies are accelerating their moves to the cloud to become more cost-efficient, agile, and innovative. More than ever, the cloud has become vital, all thanks to the flexibility, speed-to-market, pay-as-you-go pricing, and on-demand capacities it provides. Although, few companies may delay the cloud initiatives, a careful analysis, planning, and execution can make it worth investing. Many organizations are implementing cloud-first strategies and are moving towards a multi-cloud strategy. This trend highlights the need for data engineers to learn and develop cloud strategies, the way to deploy apps on the multi-cloud data lake, and discover the new cloud data engineering paradigms.
- Database Streaming
Unlike in traditional data engineering, wherein database administrators would typically load data via an ETL tool/process at regular intervals such as nightly or weekly, a streaming database may sit alongside RDBMSs to provide real-time alerts. With the rising volume and velocity of data, some technologies that once relied primarily on batch-oriented databases now rely more heavily on real-time database streaming. This can enable a speedy response to events, getting real-time alerts, real-time data analysis, and much more.
- Self-service Analytics
Self-service analytics tools allow end-users to analyze their data, develop their reports, and discover related queries’ solutions with no to minimal IT support. This can aid non-technical users to process complex data. While this may seem to be highly useful to end-users, the data engineers can also gain trust in data by creating self-service capabilities for the business users, leveraging automation & scheduling to streamline data pipelines, governing the pipelines efficiently, prototyping new data products, and discovering significant business improvements.
- Containerized Apps
This OS-level virtualization method deploys and runs distributed apps without having to actually launch on the machine thereby enabling scalability. Although the architecture doesn’t seem to be different, data engineers need to learn how to manage the containers and the best practices in terms of network, security, storage, containers life cycle, monitoring, etc and how Kubernetes and other container works.
- Edge Analysis
Unlike traditional analysis models, edge analysis emphasizes speed and decentralization as the incoming data streams are analyzed at a non-central point and near the source of data generation such as a switch, a peripheral node, or a connected device or sensor. With the increasing amount of data being processed at the edge, the data engineers will have to learn and identify the new ways of deploying, updating, and monitoring analytics at the edge along with modern technologies like digital twins to mitigate development, testing, and validation challenges.
Closing:
Data engineering makes data science more productive and the lack of it will make organizations spend more time preparing data analysis to solve complex business problems. Data engineers are often tasked to manage and organize the data, while also keeping a close eye on the trends or inconsistencies that will impact business goals. Knowing these data engineering trends can help to stay relevant in the data world.
To sum up, 2022 will see a phase where transitioning to the cloud, streaming database, self-service analytics, containerized apps, and edge analysis will continue to accelerate. Besides this, other trends like Metadata management, data mesh principles, and lakehouse systems will become mainstream and reshape the data engineering architecture in the coming time.
If you’re looking to leverage data engineering capabilities in your organization, get in touch with our experts who can uncover trends in data sets and build algorithms and data pipelines to make raw data beneficial for your organization. Let’s get started!